Bias And Fairness In Ai Algorithms Plat Ai
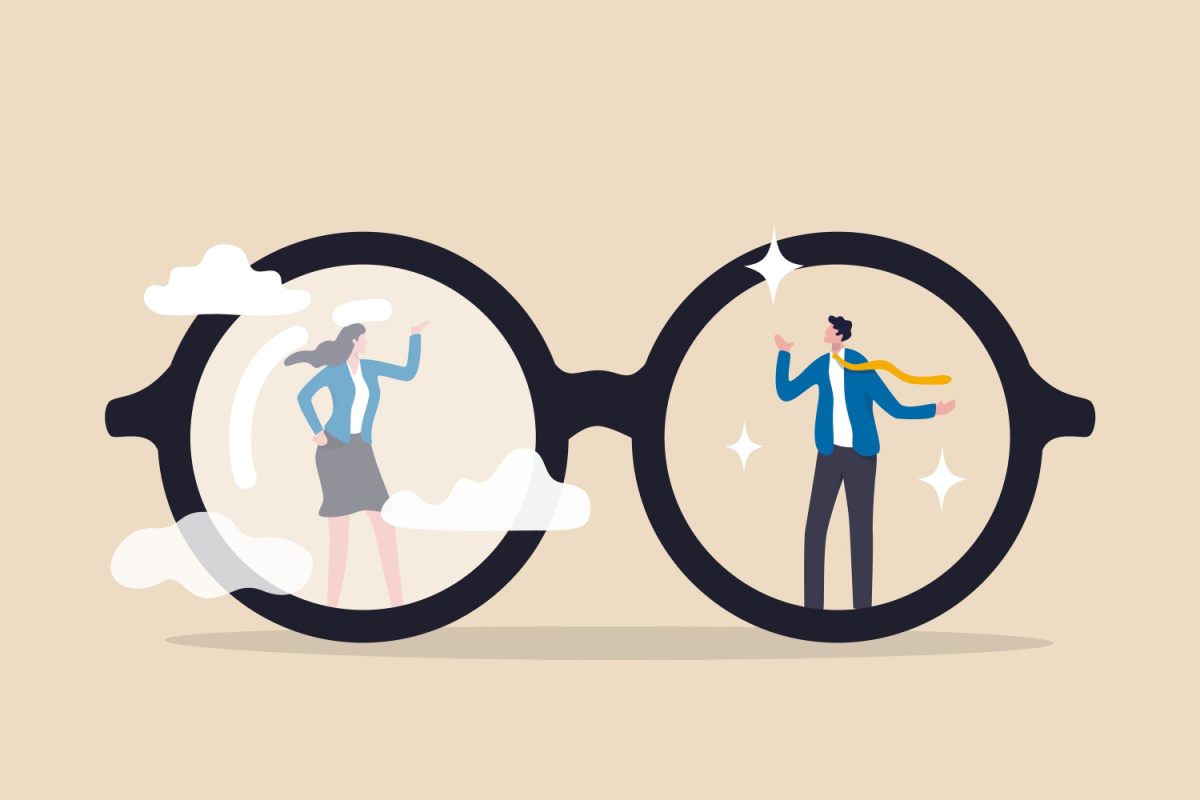
Bias And Fairness In Ai Algorithms Plat Ai Fairness in ai is a growing field that seeks to remove bias and discrimination from algorithms and decision making models. machine learning fairness addresses and eliminates algorithmic bias from machine learning models based on sensitive attributes like race and ethnicity, gender, sexual orientation, disability, and socioeconomic class. Ai's new frontier: claude 3.5's revolutionary models. october 26, 2024. algorithmic bias poses a significant threat to the ethical use of artificial intelligence. this article delves into the origins of bias within ai, its potential consequences, and the urgent need for ensuring fairness in ai driven decision making.
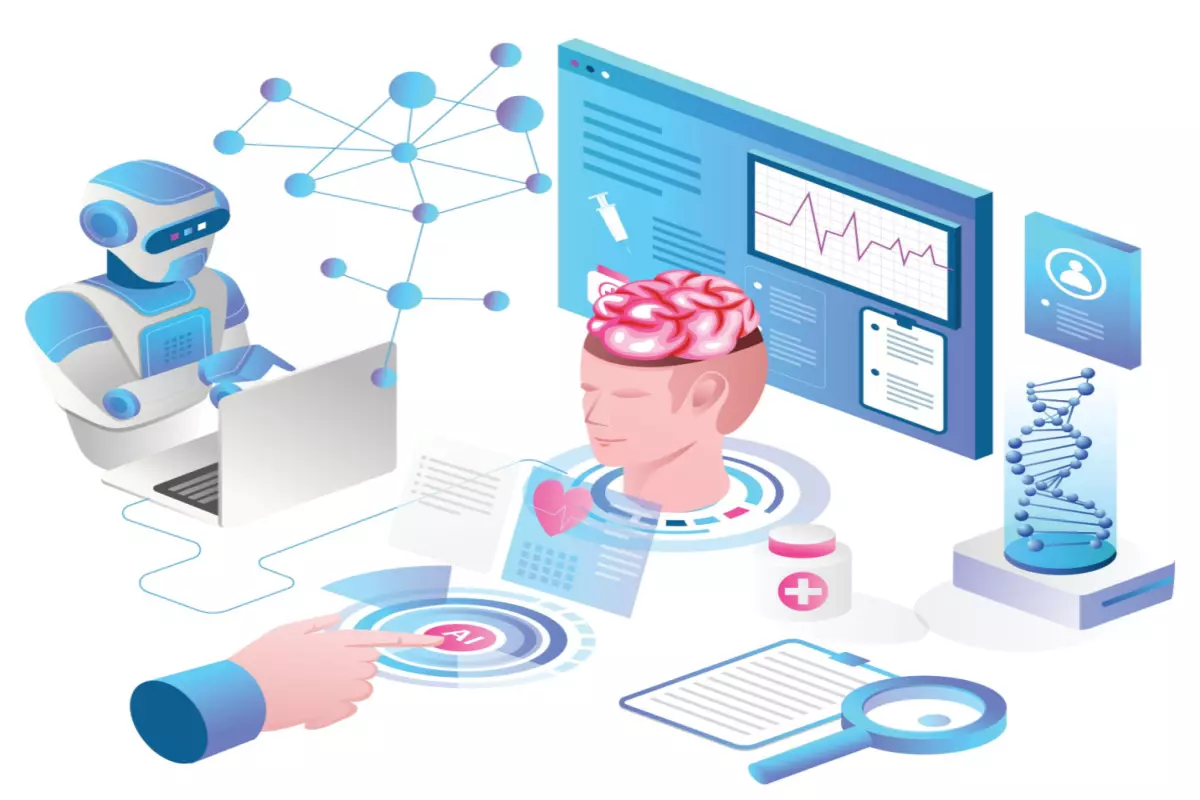
Bias And Fairness In Ai Algorithms Plat Ai Conclusion. ai bias and fairness are complex and diverse, yet they play a critical role in establishing the ethical parameters of ai systems. bias, which can come from a variety of sources, makes it difficult to make equitable decisions, but fairness acts as a beacon of ethical conduct, ensuring impartiality and inclusion. Addressing algorithmic bias has emerged as a significant concern, driving extensive research on ai fairness within both the ai community and society at large. while traditional approaches operate within constrained supervised learning paradigms, recent advancements have recognized the challenges posed by real world scenarios where class labels. Mar 24, 2021. 2. when ai makes headlines, all too often it’s because of problems with bias and fairness. some of the most infamous issues have to do with facial recognition, policing, and health care, but across many industries and applications, we’ve seen missteps where machine learning is contributing to creating a society where some. Three groups could be identified when relating bias to the life cycle model: data bias, learning bias, and deployment bias [1]. the metrics commonly used to measure fairness are associated with the comparison of privileged (pg) and underprivileged (ug), but there are also metrics to compare individuals, although they are less popular [11] .
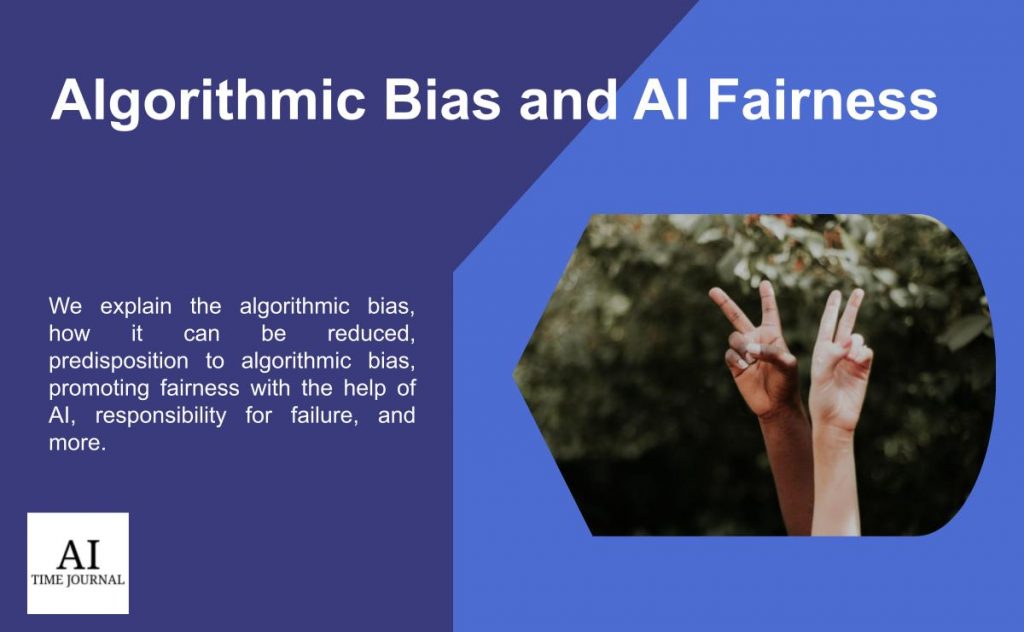
Algorithmic Bias And Ai Fairness Ai Time Journal Artificial Intelligence Automation Work Mar 24, 2021. 2. when ai makes headlines, all too often it’s because of problems with bias and fairness. some of the most infamous issues have to do with facial recognition, policing, and health care, but across many industries and applications, we’ve seen missteps where machine learning is contributing to creating a society where some. Three groups could be identified when relating bias to the life cycle model: data bias, learning bias, and deployment bias [1]. the metrics commonly used to measure fairness are associated with the comparison of privileged (pg) and underprivileged (ug), but there are also metrics to compare individuals, although they are less popular [11] . Currently, ai fairness 360 suggests all pre processing algorithms, “should be tested because the ultimate performance depends on dataset characteristics: there is no one best algorithm. This special track includes a curated selection of papers in extended form from the 1st aequitas workshop on fairness and bias in ai, held in kraków in october 2023, in conjunction with ecai 2023. ai based decision support systems are increasingly deployed in industry, in the public and private sectors, and in policymaking to guide decisions.
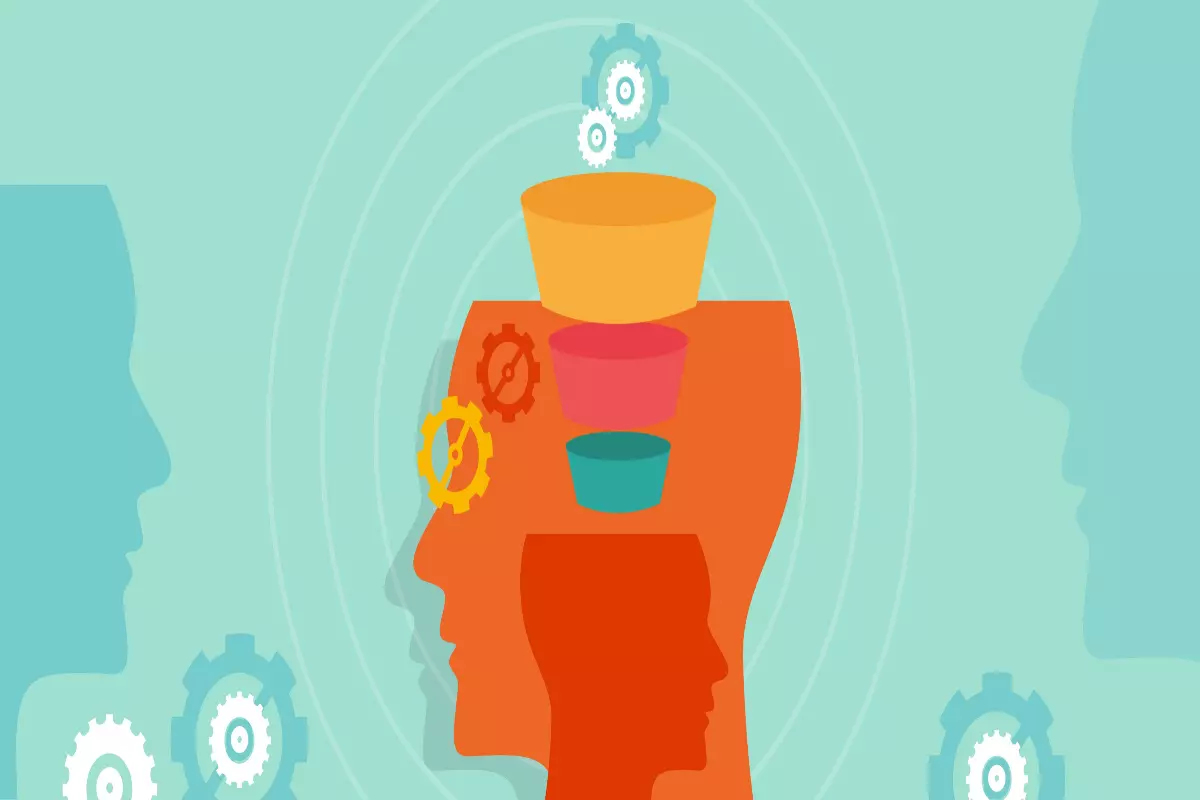
Bias And Fairness In Ai Algorithms Plat Ai Currently, ai fairness 360 suggests all pre processing algorithms, “should be tested because the ultimate performance depends on dataset characteristics: there is no one best algorithm. This special track includes a curated selection of papers in extended form from the 1st aequitas workshop on fairness and bias in ai, held in kraków in october 2023, in conjunction with ecai 2023. ai based decision support systems are increasingly deployed in industry, in the public and private sectors, and in policymaking to guide decisions.
Comments are closed.